It’s impossible to miss the current buzz around artificial intelligence, a subject that deserves our attention. It took me a while to fully immerse myself in it. At first, I was skeptical about the hype surrounding AI. It’s not the first time we’ve heard talk of its imminent arrival, when in reality it’s humans masquerading as AI.
Worried, I also took a long time before immersing myself in tools like ChatGPT or Midjourney. The fear of gradually delegating tasks to a tool and becoming obsolete, the guilt of using tools that could threaten the existence of certain creative professions. And a general mistrust of data processing.
Nevertheless, aware that ignorance about artificial intelligence won’t protect us from its impact on our lives, I finally decided to explore the subject and write this introductory article for everyone.
Two types of Artificial Intelligence
First, let’s distinguish two types of artificial intelligence. There is ANI and AGI. ANI stands for “Artificial Narrow Intelligence”, and is the most common type of AI, specializing in a single task. An AI that produces only text, like ChatGPT, or only images, like Midjourney, is an ANI.
Artificial General Intelligence, on the other hand, is an AI that can combine several tasks. For the same query, produce text, image, and sound that would respond to that query. When you think of a destructive AI like in the movie “Terminator”, it’s an AGI intelligence. But, as this type of intelligence is difficult to develop, the replacement of humans by machines will have to wait. Nevertheless, OpenAI’s mission is to create an AGI.
Our mission is to ensure that artificial general intelligence—AI systems that are generally smarter than humans—benefits all of humanity.
Machine Learning, Deep Learning and Data Science
You may know the definitions of these terms individually, but do you know how they relate to Artificial Intelligence?
Quite simply, Machine Learning, Deep Learning and Data Science are all Artificial Intelligence techniques. Machine Learning is a component of AI. Deep Learning is a component of Machine Learning. And Data Science is present in many of these technologies.
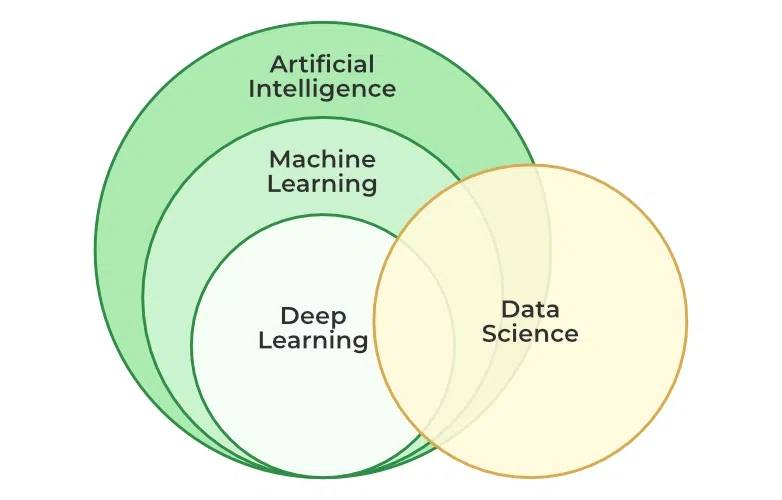
Machine Learning
Machine Learning is a field of study that gives computers the ability to learn without being explicitly programmed. This is achieved through supervised learning, with programmers providing a large set of already-transformed data and instructions. The computer must then produce an output result. Throughout the process, the machine is not guided. Instead, it is told whether its result is positive or not, so that the program can learn from its mistakes.
In unsupervised learning, the algorithm asks the computer to analyze and group unlabeled data. These algorithms identify groups of similar data without human intervention.
Their ability to discover similarities and differences between information makes them ideal for exploratory data analysis, data segmentation or image recognition.
Deep Learning
Deep Learning uses neural networks to solve complex tasks. Neural networks are so called because they are simply inspired by the functioning of the neurons in our human brain. However, these systems have not yet reached the level of our brains. There are supervised and unsupervised Deep Learning methods. Instead of using task-specific algorithms, it learns from representative examples. For example, if you want to build a model that recognizes cats by species, you need to prepare a database that includes numerous cat images.
Deep Learning | Machine Learning |
Large amounts of data | Small datasets, as long as they are high-quality |
Computation-heavy | Not always |
Draw accurate conclusions from raw data. | Careful pre-processed data. |
Takes much longer to train. | It can be trained in a reduced amount of time. |
You can’t know what are the particular features that the neurons represent. | The logic behind the machine’s decision is clear. |
It can be used in unexpected ways. | An algorithm is built to solve a specific problem. |
Deep Learning is not as dependent on human intervention as Machine Learning. Deep Learning enables discoveries to be made even when developers aren’t sure what they’re looking for.
Deep Learning is used, for example, in speech recognition, pattern recognition, image recognition, natural language processing and recommendation systems.
Data Science
Data Science is the field of study that helps extract knowledge and information from a database. This is often a data set that can be used to make business decisions.
Generative AI
Generative AI is a type of ANI artificial intelligence. Generative AIs can produce text, images, sound or video in response to prompts. ChatGPT or Midjourney are generative AIs. The power of generative AIs is to produce an “original” rendering. For example, AIs that can create faces of people that don’t exist thanks to their image database. In contrast, a non-generative AI will only produce content that already exists in its input, such as translating a sentence.
Generative AIs are a cause for concern. If an AI can create content on the same level as a human, it raises questions of content authenticity, copyright, and the value of human creativity. For sectors such as journalism, entertainment, design and publishing, generative AIs could turn their businesses upside down.
Why is AI trending now?
Artificial intelligence is not a new technology. Arthur Samuel defined Machine Learning in 1959. Artificial Intelligence became an academic discipline in 1956. AlphaGo, the intelligence that beat the best Go player, a game thought impossible for an AI to crack, beat 9-dan champion Lee Sedol in 2016. The OpenAI non-profit association was also founded in 2015.
What has boosted and propelled AI to the forefront of all tech scenes is the profusion of data available. The more data an AI model receives, the better its performance. Data is now everywhere, thanks to our use of the Internet, our smartphones, cookies, etc. AI models such as ChatGPT or Midjourney have developed the ability to fetch the data needed to respond to our queries with almost original content. If Midjourney or DALL-E manage to create images from text queries, it’s by analyzing thousands of existing images referenced on the Internet.
AI’s ecosystem
Worldwide, three American companies share the biggest slice of the pie: DeepMind (Google), OpenAI (Microsoft) and Anthropic (Amazon).
In 2015, the OpenAI foundation received a billion dollars from several investors (Thiel, Musk, YC Research…). In 2019, OpenAI received a billion dollars from Microsoft. But after the release of ChatGPT, everything accelerates, and OpenAI can finally share in the fruits of these investments. In January 2023, OpenAI will receive no less than $10 billion from Microsoft, spread over several years. All this money will enable companies to recruit the best artificial intelligence engineers and researchers. Where before, artificial intelligence was an academic discipline where the best profiles worked for universities or the public. Now they’re being hunted down and recruited at ultra-competitive salaries to join the private sector.
AI’s french ecosystem
France is not standing still when it comes to AI. On the contrary, Paris is aiming to become Europe’s artificial intelligence capital. Mistral, Nabla, Dust and Hugging Face are headed by former OpenAI, Meta and Google employees, and have secured millions in investment from funds such as Sequoia, Lightspeed, Xavier Niel and Eric Schmidt.
How will French companies try to compete with American companies? Through talent. France has been training excellent mathematicians, engineers, and scientists for years. But, until now, the best profiles went to the United States in search of better professional opportunities. With the new AI craze in France and French entrepreneurs returning to France to set up their startups, the ecosystem is keen to retain these talented profiles with better salaries. Even if average salaries will remain lower than in the US, these profiles will be more encouraged to pursue their careers in France.
“80% of our users are in the U.S., but we’re based in Paris because AI engineers are good and cheap compared to the U.S.” Alexandre Lebrun, co-founder of Nabla.
Xavier Niel has announced the creation of an open source artificial intelligence research laboratory. A laboratory that convinced former Google CEO Eric Schmidt to invest 300 million Euros in it.
Despite these investment efforts and the enthusiasm for a new technology, bad habits persist. The artificial intelligence and machine learning sector remains male-dominated. Which is a great pity when you consider that a non-diversified team is more likely to create AI with discriminatory biases. But that’s the subject of a future article.
Leave a Reply